Two-Stage Deep-Learning Classifier for Diagnostics of Lung Cancer Using Metabolites.
Publication Title
Metabolites
Document Type
Article
Publication Date
10-7-2023
Keywords
lung cancer; machine learning; metabolites; california; pni
Abstract
We developed a machine-learning system for the selective diagnostics of adenocarcinoma (AD), squamous cell carcinoma (SQ), and small-cell carcinoma lung (SC) cancers based on their metabolomic profiles. The system is organized as two-stage binary classifiers. The best accuracy for classification is 92%. We used the biomarkers sets that contain mostly metabolites related to cancer development. Compared to traditional methods, which exclude hierarchical classification, our method splits a challenging multiclass task into smaller tasks. This allows a two-stage classifier, which is more accurate in the scenario of lung cancer classification. Compared to traditional methods, such a "divide and conquer strategy" gives much more accurate and explainable results. Such methods, including our algorithm, allow for the systematic tracking of each computational step.
Area of Special Interest
Neurosciences (Brain & Spine)
Area of Special Interest
Cancer
Specialty/Research Institute
Oncology
Specialty/Research Institute
Pulmonary Medicine
DOI
10.3390/metabo13101055
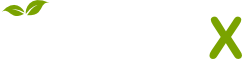
- Citations
- Citation Indexes: 1
- Usage
- Abstract Views: 3
- Captures
- Readers: 4
- Mentions
- Blog Mentions: 1
- News Mentions: 1